
Why using technology is important for data management
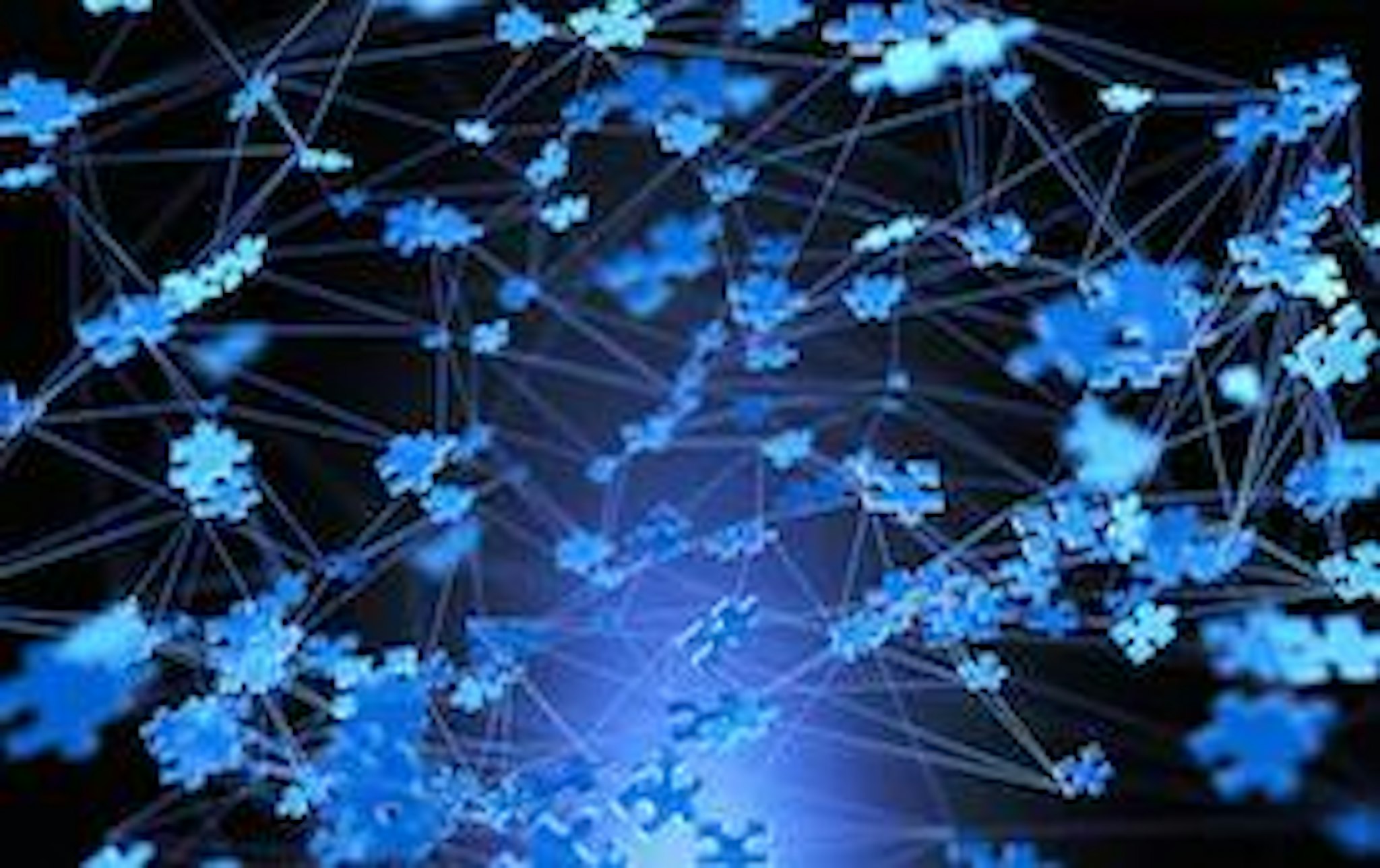
The volume of data we create is ever expanding. Every time we conduct a search, complete a purchase, visit a website or comment on a post, we add to that vast ocean of information. As we all know, this information can be a valuable asset, but only if we know how to tap into and extract the bits that are relevant for our use.
It is a well-recognized fact that the volume of data has increased far faster than most companies' ability to process it. According to a recent study, this glut of data has crippled some executives, with 61 percent of managers reporting an information overload at their workplace. This overload led more than 50 percent of them to ignore current data in their decision-making processes simply because they did not have the means to transform that data into actionable information. This leads to the question: What decisions could your company make if you had all of the information you needed?
Data management systems provide the key to utilizing data in its many, various forms.
Strategic implementation of data management technologies can help your company achieve:
- Improved organizational consistency
- Increased productivity
- Greater collaboration and communication
- Faster, more knowledgeable business decisions
Failure to take advantage of the wealth of opportunities data management technology provides could place your company at risk of:
- Conflicting information and analysis
- Decreased productivity
- Organizational waste
- Missed opportunities
This post will explore some of the many sources of data, describe some of the techniques used to capture and describe that data, and provide real world examples of how companies are using data to expand their customer base.
Where is the data coming from?
Data is created by a vast multitude of different sources. Data analysts tend to define data as either structured or unstructured.
Structured data is highly organized information that can already be easily indexed, uploaded into databases, and detected by search operations or algorithms. Structured data usually consists of objective, numerical information that does not require interpretation.
Some sources of structured data include:
Transactional Data
- Point-of-sale transactions
- E-commerce or online purchases
- 'Behavioral' transactions, such as clickstream data
Machine and Sensor Data
- Data generated by functional devices, such as network-connected home appliances
- Smart utility meters
- Monitored processes of factory machinery
Mobile Data
- Data generated or recorded by in-app transactions
- Updates in status or locations
- Webserver logs
Unstructured data is a kind of catch-all phrase describing data that resists easy indexing and may not conform to existing database organizations. Unstructured data is human-generated and often language-based, and as such, tends to be less focused and harder to categorize.
Examples of unstructured data include:
- Information contained in emails
- Audio and video files
- Blogs and wikis
- Postings on Twitter, Facebook, Instagram and other social media platforms
Structured data tends to describe moments in time that can be distinct and accountable, i.e., when and where a purchase took place, or at what point a machine reached a certain level of production. Unstructured data tends to reveal more about opinion, emotion, and relationships between customers and products.
How do you sift through the data?
Now that we have identified some of the sources of data, what techniques are available for transforming it into actionable knowledge? The most common techniques are data mining and text mining.
Data Mining
Data mining processes review large amounts of data searching for consistent patterns or relationships. They then attempt to validate these potential patterns by applying them to new subsets of data. The two main tasks of data mining technology are the creation of descriptive and predictive powers. Descriptive powers attempt to find interesting, interpretable trends in the available data sets. These trends create a picture of what exists now. The predictive powers use that information to extrapolate unknown values into the future.
Some of the more fundamental techniques used in data mining include:
- Association: Making a correlation between two items to determine patterns. For example, in a study of purchasing habits, you may note that people often buy cream and coffee together, thus creating an association between them.
- Classification: Defining multiple attributes used to identify an item, a product or a customer. For example, you could classify a potential customer pool based on age, income and zip code.
- Sequential Patterns: Used to analyze long-term data to find regular occurrences of similar events. This can be useful when noting that customers tend to buy or search for similar products at particular times of the year. For example, this data may help you notice that as summer approaches, more customers search for vacation homes.
Want more examples of how data mining helps companies increase their income? Think of Amazon's ability to cross-sell and up-sell items. Using the descriptive power of data mining, they know what their customers have bought in the past. By utilizing the predictive power, they can suggest other products and perhaps garner more sales in the future.
Text Mining
Data mining is typically limited to numerical data, but as we have seen, critical business information is often contained in text documents. This is the unstructured data alluded to above. As the name implies, this data is less predictable, more amorphous and harder to deal with. Text mining technologies analyze multiple documents, zeroing in on key concepts, phrases or word usages.
Some key techniques of text mining include:
- Entity Extraction: Identifying and classifying key elements, such as people, places or companies within a text into predefined categories. This allows an analyst to review a quick, structured representation of the document's contents.
- Sentiment Analysis: Identifying and categorizing opinions expressed in a piece of text in order to determine the writer's reaction to a topic is positive, negative or neutral.
Text mining is one way of exploiting the vast amount of unstructured data produced by consumers. Trying to evaluate the effectiveness of your latest email blasts? Curious to hear the buzz generated by your company's Twitter posts? Text analysis processes help you gauge your customers' opinions and desires, and may help clue you in to potential complaints or misfires.
Feeling Overwhelmed?
Do you feel crippled by the glut of data in your workplace? Do you worry you might be missing out on valuable business information that could transform your day-to-day processes? Blueprint's entity management systems are here to help. Trust Blueprint to help organize, analyze and utilize the data your company depends on for faster, more knowledgeable decision-making.